Earlier Alzheimer's detection anywhere in 10 minutes
AI-enabled software for detecting subtle cognitive impairment and Alzheimer's neuropathology, based on how people speak.
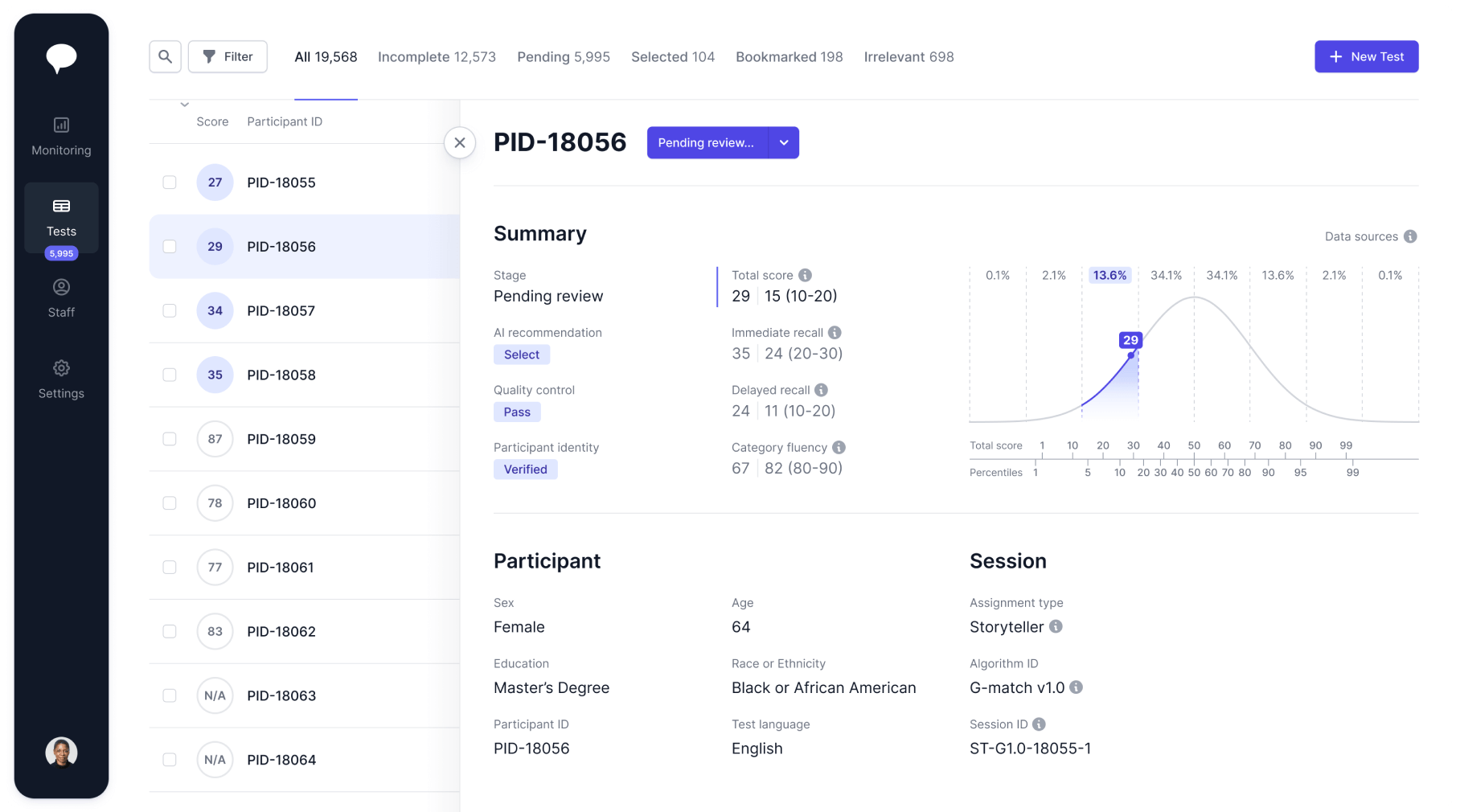
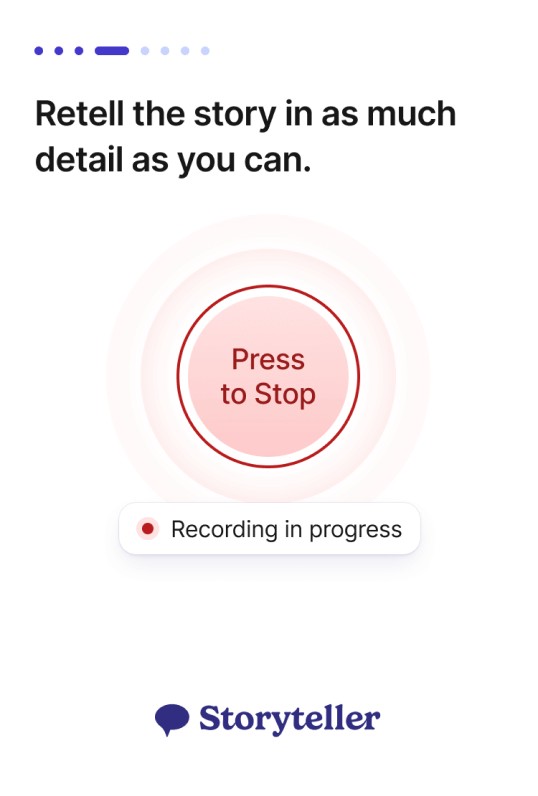
Our algorithms are finding more early-stage Alzheimer’s patients faster for 50+ institutions:
Scaling access to early detection of Alzheimer’s disease
Early detection is needed for effective treatment but <1% have access today. Speech-based testing can offer scalable access for anyone anywhere. We’re supporting early detection in underserved communities across 50+ medical centers together with the Alzheimer’s Disease Neuroimaging Initiative.
Novoic Storyteller
Speech-based cognitive testing that is real world ready
Automated assessment
Our audio-verbal assessment is a fully automated experience, tested on thousands of users. It’s self-assessment that simply works.
Low-burden, high usability
Works on any smart device
Maximizing data integrity
Better algorithms, better results
Earlier detection of decline
Our models accurately detect mild cognitive impairment, including subthreshold impairment in preclinical Alzheimer’s disease.
Differential diagnosis of neuropathology
Advanced AI models
Gold standard validation
We’ve run our own clinical trials in biomarker-confirmed prodromal and preclinical Alzheimer’s, combined with diverse datasets, to make our next-generation algorithms more predictive, more robust and more equitable.
The AMYPRED clinical studies have evaluated speech biomarkers in the earliest stages of biomarker-confirmed Alzheimer’s disease
Real-world datasets in diverse communities improve robustness to demographic variance.
Automated assessments and rapid analysis outputs inform patient selection in over 50 medical centers in the US.
Novoic Dashboard
Manage digital screening and monitoring at scale
AI-assisted triage
AI-enhanced recommendations support screening and inclusion. Triage is configurable from fully manual to fully automated.
Easy to use
Simple to implement
Monitoring at scale
Software you can trust
Our highest priority is ensuring your data’s privacy, security and quality. Our approach tightly integrates good software development practice, good clinical practice and good quality management.
Integrated HIPAA/GDPR compliance and automated backups guarantee the privacy and integrity of your data throughout our ecosystem.
Gold-standard security protocols built into the foundation of our software protect your data against cyber risk.
Software development is led by our digitally native QMS, designed for compliance with evolving medical software quality standards.
Join 50+ top institutions
Tools to give your team superpowers
Set up a time with us and see our software in action
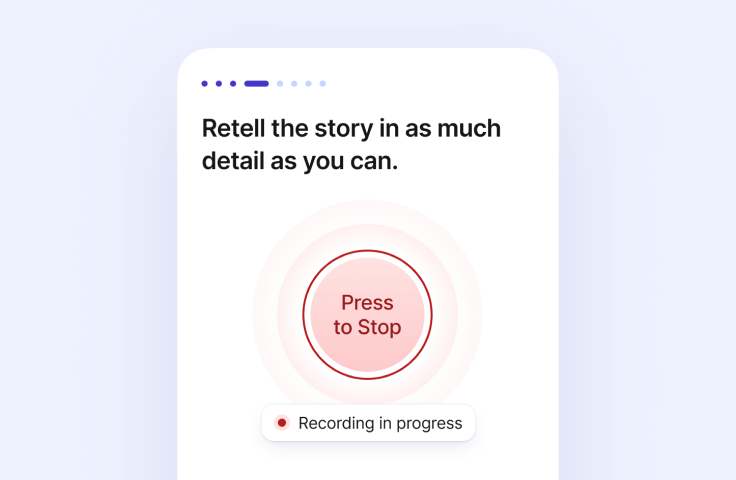
Storyteller
Leverage online automated testing to radically scale access to patients. Use state-of-the-art AI-based speech biomarkers to detect patients earlier with higher confidence.
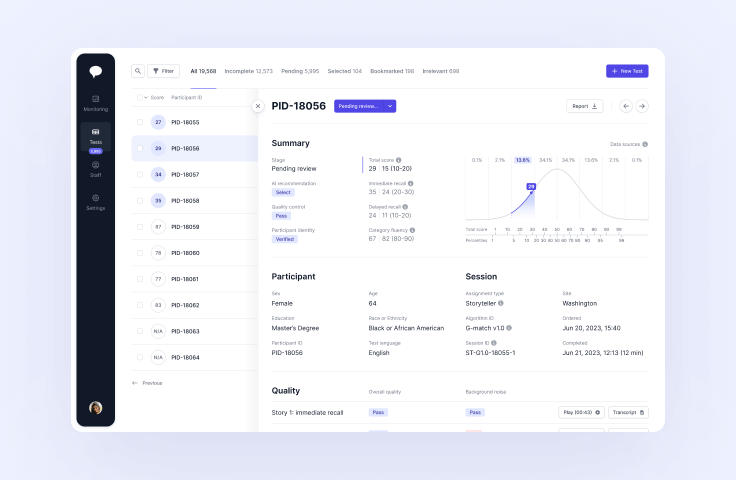
Dashboard
Find the right patients faster with AI-supported screening. Oversee and manage your whole patient funnel across and within research sites. Explore your data with detailed and flexible analytics.
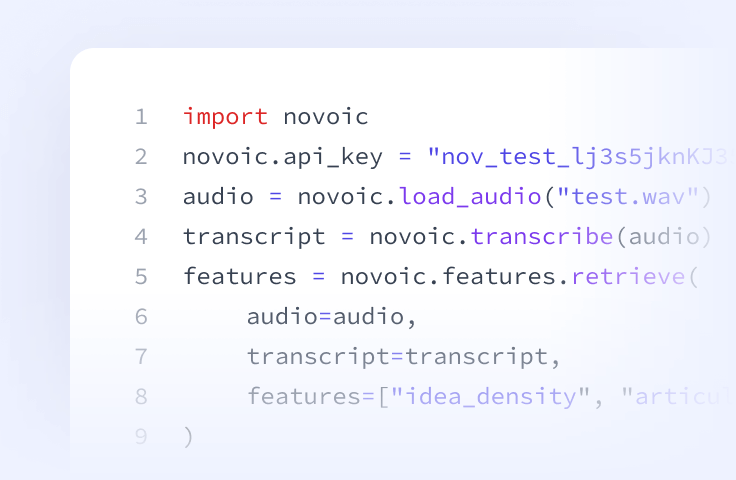
Speech API
Prefer to build it yourself? Access our speech processing, ASR, automated quality control and AI-based speech biomarkers directly via API.